*changes may apply
Artificial Intelligence and Deep Learning Development Syllabus
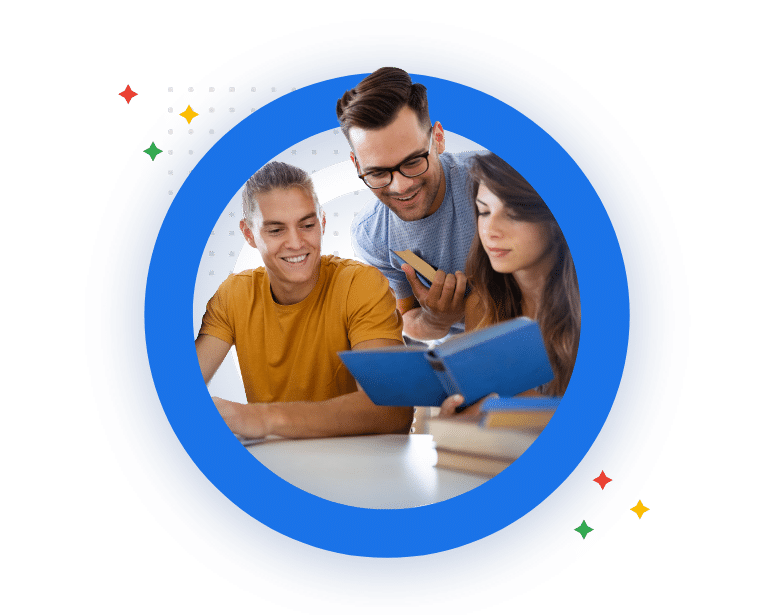
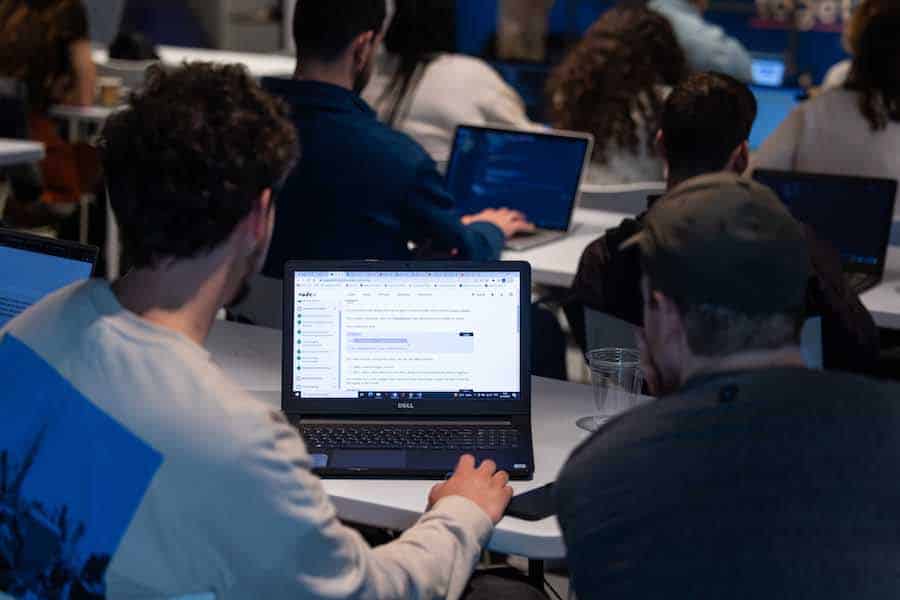
Introduction
- Gemini, ChatGPT and other impressive artificial models.
- Human and Artificial Intelligence, Turing test, brain, neurons, synapses
- Brief history of AI
- Terminology: Machine Learning (ML), Supervised vs. unsupervised learning, Neural Networks (NN), Deep Learning (DL), NLP, LLMs, Reinforcement Learning (RL).
- Typical applications of AI. How to join the revolution?
- Imbalanced Class
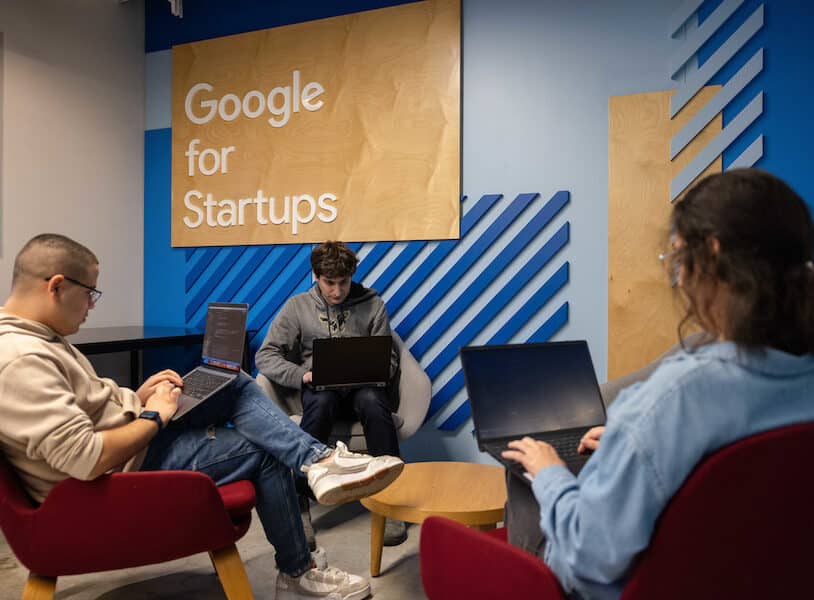
Before AI - A quick review of some classical models
- Basic statistics
- Linear regression
- PCA
- K-means
- Decision Trees
- Approximate Nearest-Neighbors
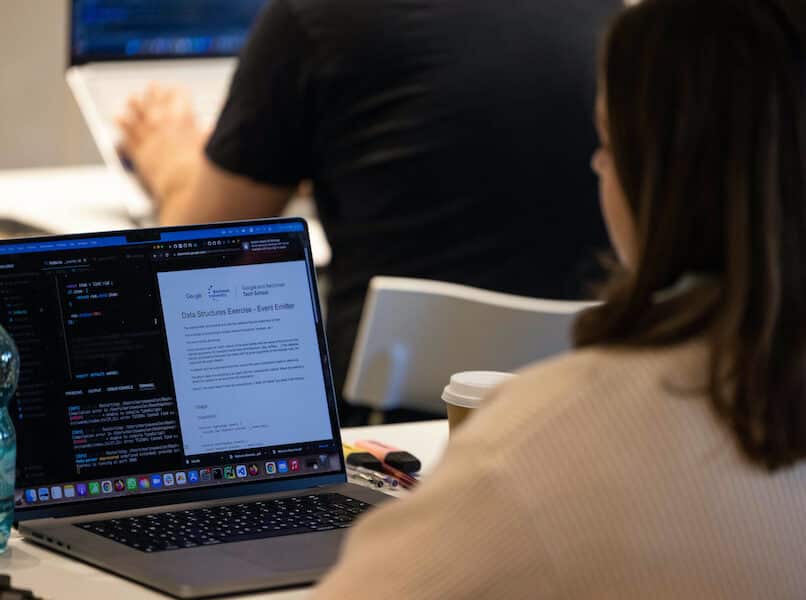
Deep Learning
- Main models and methods. Training, testing and validation.
- Using Python Notebook and Colab.
- Main AI training tools: Keras and TensorFlow by Google, Pytorch.
- Hardware and software infrastructure: GPUs, Cuda, Dockers.
- Relevant python libraries: NumPy, Matplotlib, Pandas.
- Your first Neural Network model: Detecting patterns in tabular data.
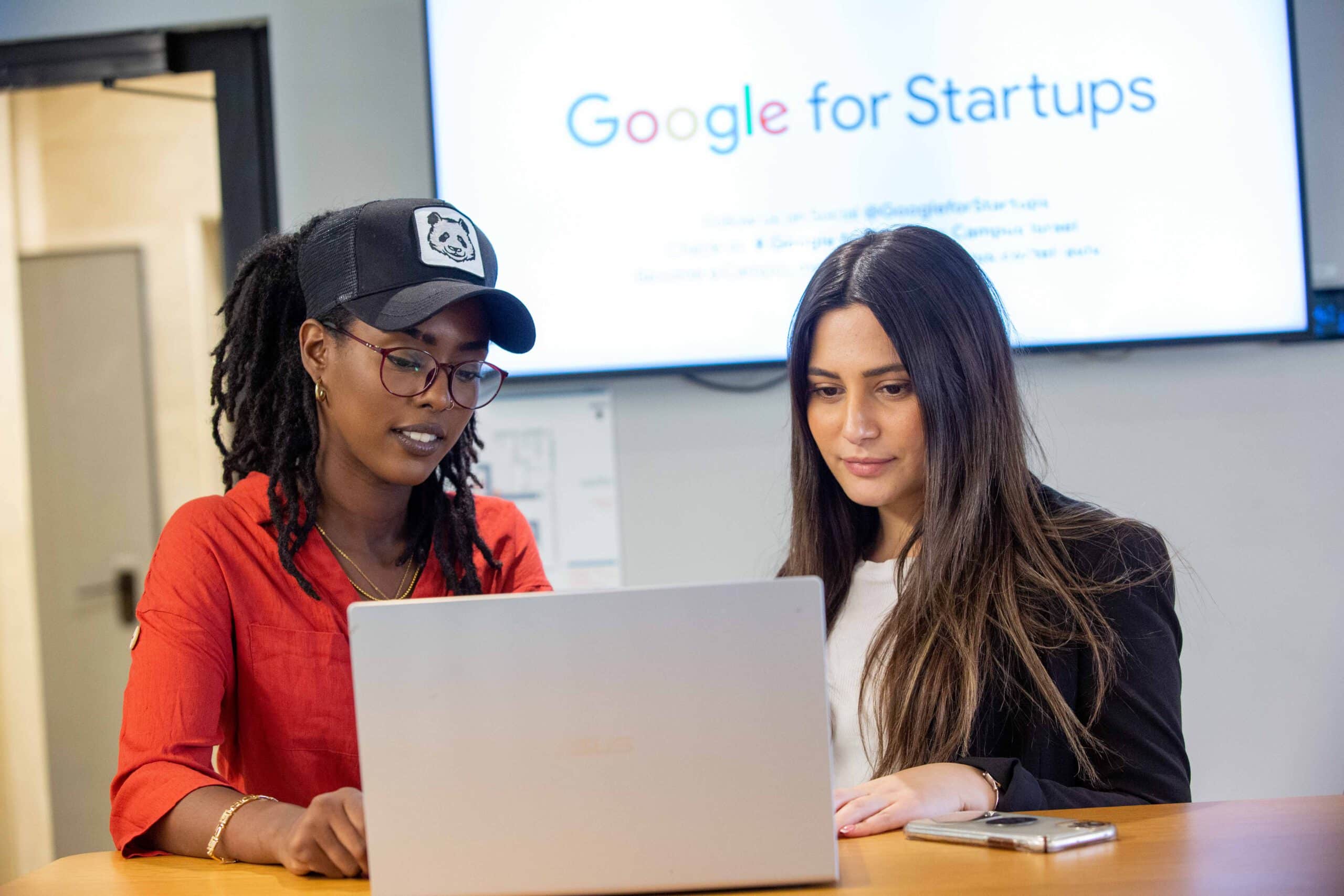
Computer vision and Convolutional Neural Networks
- Visual tasks: classification, detection, and segmentation.
- Why is Machine Vision difficult?
- NN building blocks and layers: single neuron, convolutions, pooling, fully connected layers, normalization, activation functions, loss.
- Learning the model weights: the back-propagation algorithm.
- NN architectures: feed-forward, recurrent, encoder-decoder, Siamese.
- Practical open-source vision NNs: Resnet, Yolo, Clip, SAM.
- Computer Vision mini project: detecting objects in images
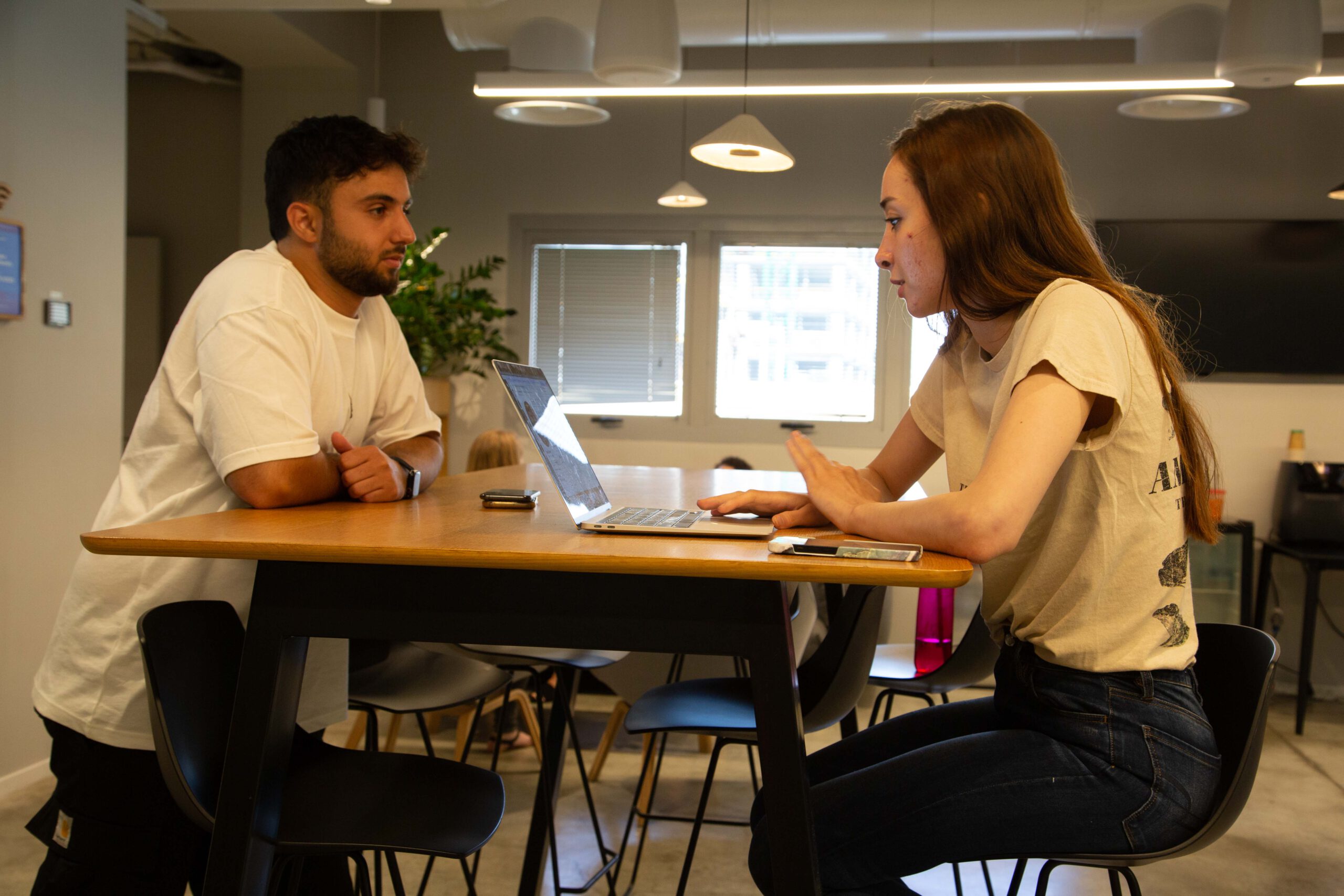
Natural Language Processing (NLP) using Transformers
- Large Language Models: capabilities and limitations.
- Using LLM services in your application: Google Vertex AI and Gemini APIs
- Training Large Language Models (LLMs) and fine-tuning chatbots.
- Attention mechanism and the Transformer architecture.
- Applications: summarization, content creation, virtual assistants, translation and transcription, Sentiment Analysis.
- NLP mini project: using LLM APIs for business use case.
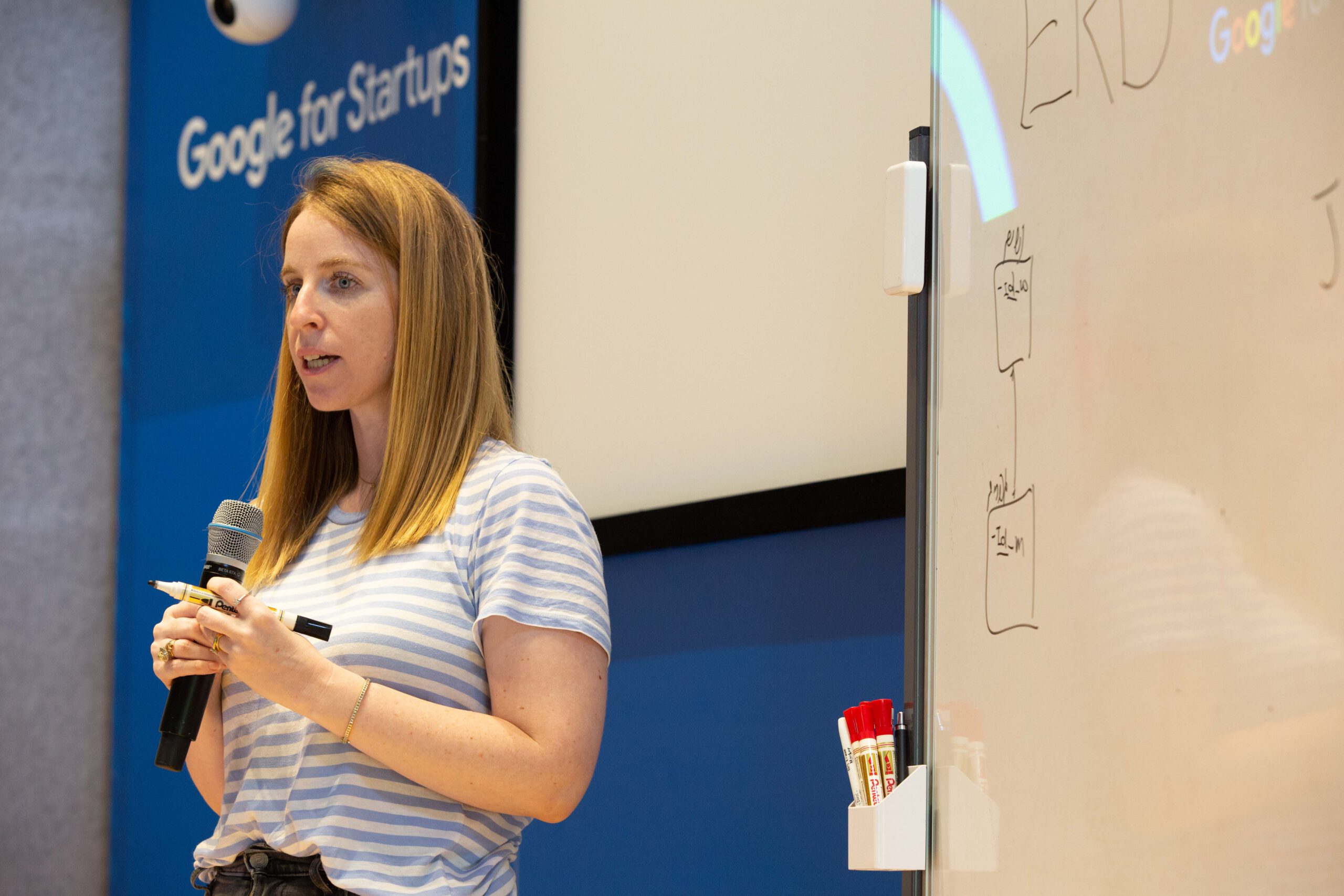
Deep Reinforcement Learning (RL)
- Introduction: reinforcement learning vs. non-interactive supervised learning.
- Methods: Q-Learning and Deep Q-Network.
- Applications: control and robotics, automated driving, trading and finance.
- Reinforcement learning mini project: gaming.
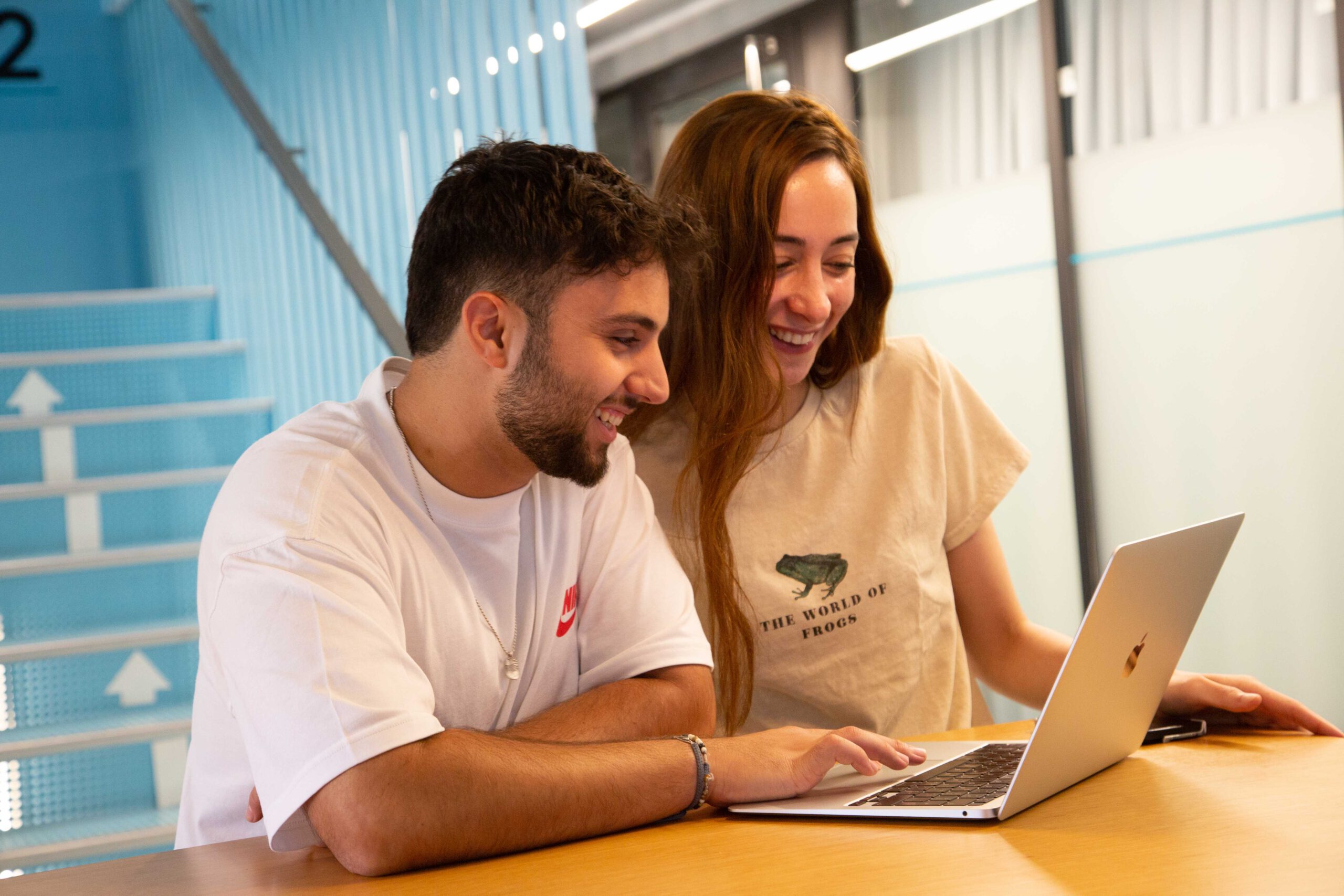
Other applications and trends
- Time series forecasting in healthcare.
- Recommendation systems.
- Cyber security and authentication.
- Generative Adversarial Networks (GANs).
- Multimodal Generative AI: image and video generation from text.
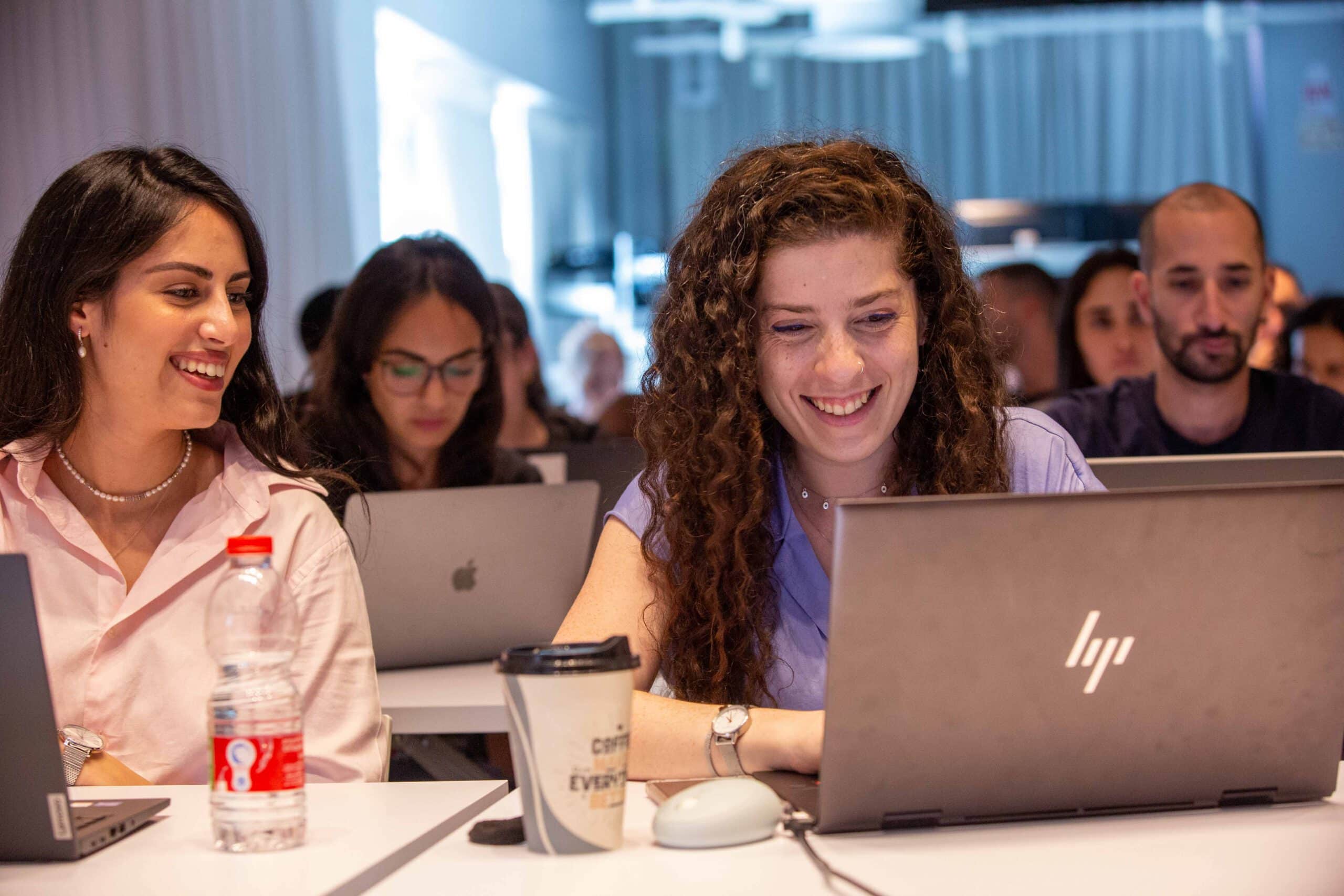
Real-life AI projects in the industry
- Choosing an open source to start with.
- Handling data: collecting, filtering, cleaning, augmenting, preprocessing.
- Validation, testing and measuring the model quality.
- Using TensorBoard: Visualizing the training process, controlling convergence and overfit.
- Using TensorBoard: Visualizing the training process, controlling convergence and overfit.
- Experimenting architectures, loss functions and hyper-parameters.
- Experimenting architectures, loss functions and hyper-parameters.
- Improving model speed: optimization, models search, pruning, distillation.
- Deploying your AI models for smartphones and edge devices with TF Lite.
- Deploying your AI models on Google Cloud Platform for scale and stability.
- AI development lifecycle with Google Cloud tools.
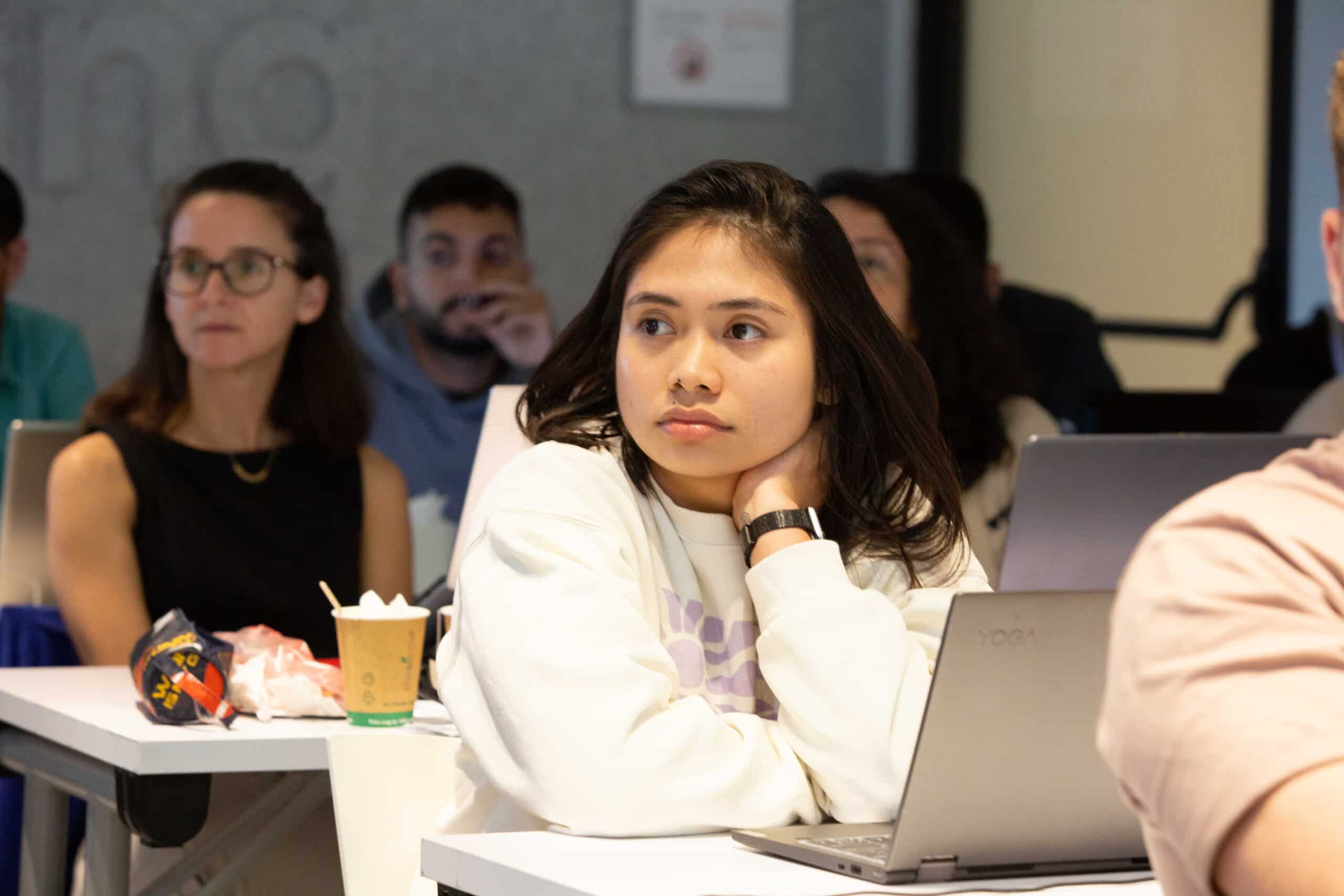
Responsible AI
-
- Personal, social, and economic implications
- Who is responsible? legal aspects
- Being fair and reducing biases
- Data privacy, compliance, and security
- Explainable AI
- Dealing with deep fake
- Who owns the data, the models, and the code libraries?
- Dangers and opportunities for mankind
- Towards synergy of mankind and AI
- Retrieval Augmented Generation (RAG)
*Changes may be applied in the Syllabus